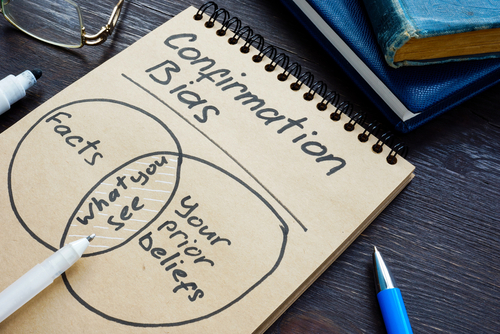
A Story
On Friday night, I had dinner with a LinkedIn contact. (As I travel, I post where I am going, and if someone reaches out for dinner or lunch, I say, “Why not?”) So, I sat across from a stranger on a cold winter night, the only thing we had in common was our experience in supply chain planning. I love hearing others’ perspectives. I started with, “How can I help you?”
As I dipped my spoon into some scrumptious chestnut soup at a great restaurant, my companion asked, “With the advancements in optimization and self-learning, aren’t we close to having self-driving supply chains?”
Shocked and trying not to drip the soup on my new dress, I asked, “Why do you believe this?”
He replied, “Aren’t we close to having self-driving cars? And won’t the supply chain follow suit?”
Before responding, I closed my eyes and thought about a recent conversation with Bruce, my prior boss. Bruce, one of the most intelligent people I have ever met, is deadlocked in a race against time to build a self-driving vehicle. As a developer working on the braking software of an automatic car for the past eight years, he constantly tells me that it is not easy, and all of the estimates are waaaay too positive.
So, I smile, catch my dinner partner’s eye and ask, “In our prior conversations, you mentioned the lack of clarity on the definition of supply chain excellence in your current deployments and how this is a barrier to implementing supply chain planning properly. The rules of the road of the supply chain are clear, yet we struggle to develop a self-driving car. While optimization continues to be deeper and more valuable, organizations aren’t clear on desired outcomes and how to make functional trade-offs. A manufacturing leader’s perspective differs from that of a logistics business leader. Remember that the supply chain is a complex non-linear system that cannot be solved by local functional optimization. As a result, better optimization engines into existing technology taxonomies is not the answer.”
Reflection
As I returned to my hotel room, I thought about this dilemma. The headline news that day was Theranos’ once-Silicon Valley star Elizabeth Holmes, 38, sentenced to over 11 years in prison by a United States District Court for defrauding investors of her firm to revolutionize blood-testing technology. I thought back to the press releases I wrote at prior supply chain technology companies, which only had a glimmer of truth. The supply chain planning industry is fraught with big claims with little substance. Self-driving supply chains are an example.
The self-driving supply chain is a distant dream. Most supply chain consultants and technologists struggle with confirmation bias.
The facts are clear. On the technology front, optimization is deeper, and the evolution of artificial intelligence enables more flexible sensing and modeling. The supply chain is growing in importance as more and more companies struggle with the extremes of disruption.
However, the prior beliefs of functional efficiency delivering supply chain excellence linger. The focus on inside-out processes tightly integrated to transactional data is a barrier. Most business leaders lack the understanding of data and process latency that creates supply chain black holes.
Many consultants struggle with the confirmation bias that deeper optimization plugged into existing planning systems is the stepping stone to self-driving or hands-free planning. Nothing is farther from the truth. A “digital brain” layered on top of functional and localized optimization throws the supply chain out of balance.
Confirmation bias
Confirmation bias is the tendency to search for, interpret, favor, and recall information in a way that confirms or supports one’s prior beliefs or values. People display this bias when they select information that supports their views, ignoring contrary information, or when they interpret ambiguous evidence as supporting their existing attitudes.
Wikipedia
What Is A Supply Chain Organization?
A supply chain is not a supply chain. Organizations come in many shapes and sizes. There is no magic ball on design: the organization’s reporting structures vary by culture and size. As shown in Figure 1, the reporting relationships vary.
Figure 1. Supply Chain Reporting Structures
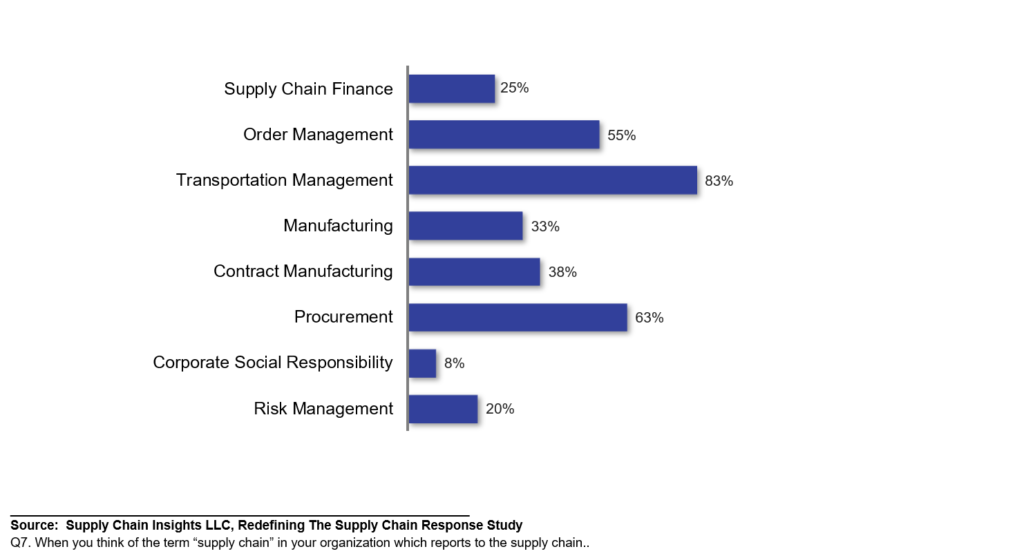
Companies having the teams from source, make, and deliver reporting to a common leader tend to be the most balanced, with the smallest gaps between operations and commercial teams. The organizations with the smallest gaps, as shown in Figure 2, drive growth faster while outperforming peers on operating margin.
Figure 2. Organizational Gaps
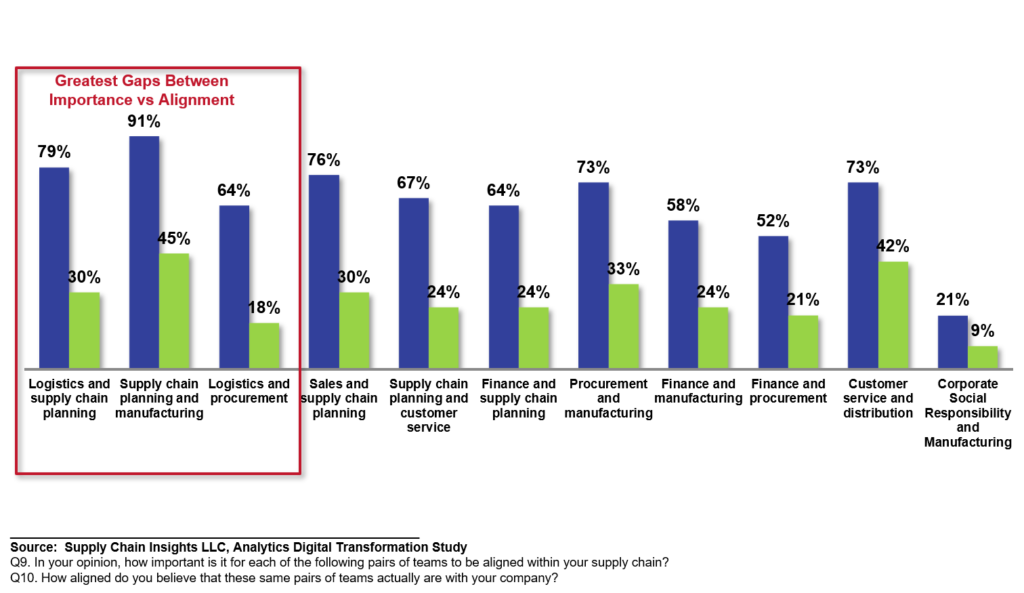
How to Drive Improvement?
With so much promise amid the intense hype, how do companies make progress? Start by analyzing something everyone in the organization can agree on: order fulfillment reliability.
The methodology is simple. Take a period—a year, six months, or a quarter—and analyze order-line discrepancies. Form a cross-functional team and ask:
- Why did the company short orders? Identify the patterns.
- Why are orders not shipped on time? Identify the routine issues.
- For each issue in the customer service analysis, ask, “When did we know? And, what did we know at the time of the decision?”
- Use this information to understand the organization’s black holes due to data and process latency. Map the data and process latency across the organization.
As you do this mapping, ask yourself five questions:
- Where are optimization engines not tethered to with decisions operating at the speed of market shifts? For example, are you using actual lead-time data? Or have you set it and forgotten it in the evolution of planning systems?
- What data is available to teams at the time of decisions? Then contrast what data should be available at the time of a decision.
- Were the plans feasible? Did the plans reflect all of the constraints? What do after-action reviews reveal?
- Are the plans executed? Measure schedule adherence in manufacturing, time for purchase order acceptance in procurement, on-time delivery for inbound materials, and first-ass tender in logistics.
- Is there data in the organization that should be used but is not?
Next Steps?
Armed with this analysis, you are ready to take the next step. Accept that existing advanced planning solutions (APS) are not sufficient. As shown in Figure 3, map the demand flows and build planning master data systems to support conventional advanced planning systems.
Then take the journey one step further, build a decision architecture to enable continual design and refinement of models to enable what-if analysis and bi-directional orchestration to eliminate the black holes while delivering a flexible what-if modeling capability.
Figure 3. Building Flexibility Using Current Advanced Planning Solutions as a Core
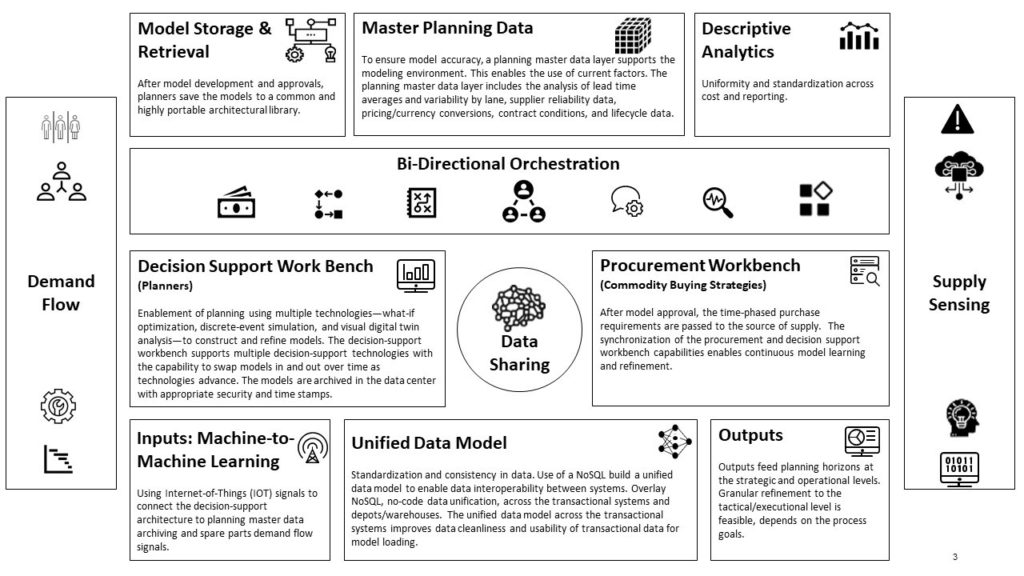
A decision-support architecture enables collaborative planning across organizations, enabling 1000s of planers to develop and modify plans. Using in-memory modeling and learning engines improves decision-making while streamlining communication to improve procurement and logistics decisions.
Redefining the cycle from plan, do, check, and adjust (PDCA) to sense-design-plan-orchestrate-act-do-learn improves the alignment for readiness. With heightened variability in lead times, supplier, labor, and transportation constraints, building a decision support framework improves accuracy and alignment on the feasibility of the best plan.
The decision-support architecture, composed of many elements, can be configured over time. A rule of thumb is that a decision support architecture is 20-30% of the cost of a transactional system with a deployment of 12-24 months. The longest element in a project plan is the redefinition of work.
The framework ideally sits within a hosting center like Azure DoD Hosting Data Center with Azure Bursting Capability details (to support large-scale but highly variable resource needs for scenario analysis). Save models with net-change capabilities using JSON or delimited text on a common, highly portable, and efficient scenario architecture.
Then accept that there is no central place to buy this architecture; instead, to accomplish this journey, you will need to cobble together multiple systems—design modeling from AIMMS, Gains, Coupa, or OMP; a unified data model from Onera, UCBOS or Microsoft, demand and sourcing sensing from multiple companies, and build a planning master data layer to improve the actionability of modeling.
Wrap-up
This architecture is not a self-driving supply chain but addresses the many limitations of existing planning architectures.
After you do your research, consider the implementation of layers of supporting architecture to help drive better decisions. I think you will be surprised at the limitations of current deployed systems because we do not feed the engines relevant data at the speed of business. The engines are getting stronger, but we cannot take advantage of the opportunity because of the limitations of original planning deployments. Don’t let confirmation bias stand in your way.
Happy Thanksgiving to those in the United States. I give thanks for the over 330,000 readers I now have on Linkedin.