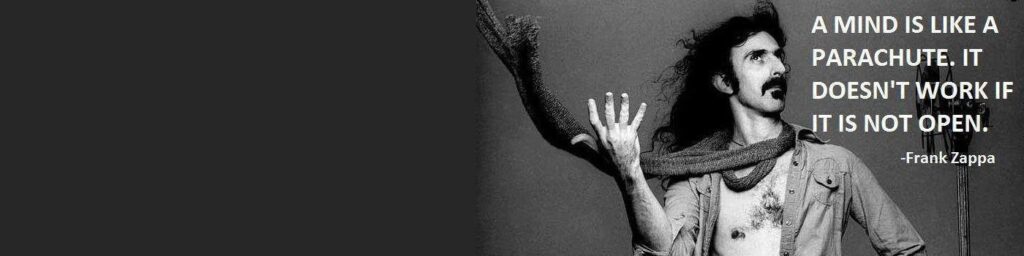
Traditional supply chain planning was defined by the theory of constraints and the Deming Wheel of Plan, Do, Check, and Act (PDCA) philosophies. The PDCA cycle, advocated by Deming in the 1950s, is based on the scientific method of proposing a change in a process, implementing the change, measuring the results, and taking appropriate action. This is the world of best practices.
As I write about the need to rethink how we make decisions with new forms of technology and the definition of a good decision, I turn to the Cynefin model advocated by my friend Trevor Miles. (Say a prayer for Trevor as he battles throat cancer.) The more I use the model, the more I like it. I stole Frank Zappa’s image from Trevor’s LinkedIn profile in honor of Trevor. I hope he gets well soon.
Driving Open-Minded Thinking
Open-minded thinking is hard for people who believe that they are experts. I find many “experts” in the industry. Still, few can answer the question of how to improve decision-making with technology and the definition of a good decision. If they do respond, the most common answer is improving forecast error, customer service, and reducing cost without reflecting uncertainty.
As we consider the possibilities of technology’s evolution, I find it helpful to change my paradigm from linear processing of information from demand to supply in the Theory of Constraints model built on PDCA (today’s philosophy of the model in traditional constraint-based model termed supply chain planning) to consider how we can improve decisions in a more uncertain world using the Cynefin Model outlined in Figure 1.
Figure 1. Cynefin Model of Making Decisions
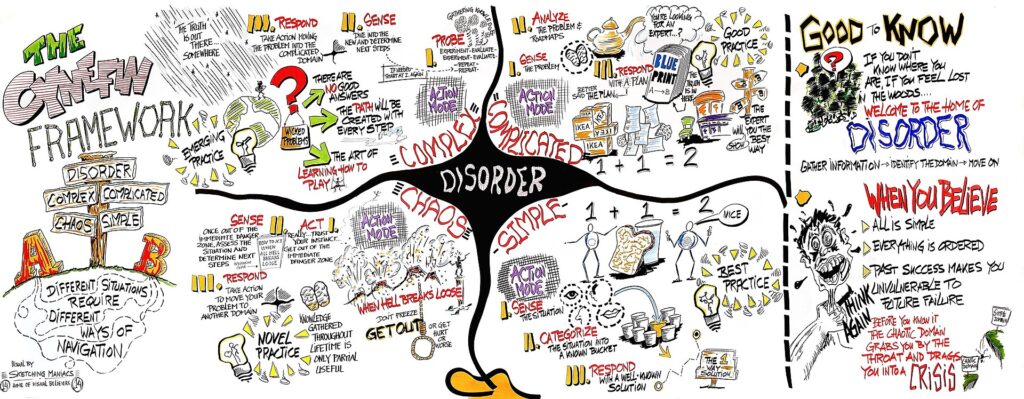
Simplistically, the underlying paradigm in the Cynefin model is that different situations require different ways of navigating and that you will never wholly eliminate the disorder. Let me explain the model counterclockwise.
- Chaos. The focus needs to be on Acting, Sensing, and Responding. Organizations need to be open to considering novel practices amongst chaos and turmoil. For example, in early COVID periods, should toilet paper be delivered directly to stores with redesigning the middle mile while sensing COVID sewage levels? Should satellite images and hotel admission rates drive consumer product distribution patterns for diapers in the fire-torn areas of LA? For example, Waffle House openings in the southeastern United States are an essential signal for hurricane recovery to align distribution policies.
- Complex. The focus is on emerging practices with a focus on Probe. Sense. Respond. The deployment of digital twins to model and simulate supply chain strategies at an executive level is a possibility to consider here. These models, unconstrained by IT batch jobs, allow business leaders to understand the potential of the supply chain as a complex, non-linear system with trade-offs and constraints before establishing policies for new product portfolios, shifting business models, or geographic expansion. One of my favorite examples is using e-commerce to test and learn consumer preferences before the launch of brick-and-mortar strategies in retail. I helped a manufacturer of men’s underwear grow its market share by testing price points and assortment on Amazon before the launch in brick-and-mortar stores. The demand latency of an e-commerce order is hours and days as compared to the traditional retail replenishment demand latency of weeks and months.
- Complicated. Moving counter-clockwise around the model, the focus is on Sense. Analyze and Respond. For me, this is the evolution of outside-in planning models that focus on a redefinition of market signals to drive bidirectional orchestration.
- Simple. This is the world of traditional supply chain planning, which focuses on sense, categorize, and then responding.
My Favorite AI Use Cases
So, why do I share this model before presenting my favorite AI use cases? I firmly believe that inserting new forms of analytics into traditional supply chain planning is not worth the trip. Instead, we need to rethink work and redefine processes. There are a variety of models, but they have moved past the theory of constraints and PDCA to fully realize the potential of new forms of analytics. As you do, constantly ask your team, “What is a good decision? How do we know if we made a good decision?” If the answer is to reduce demand error and improve cost and customer service, push the team to think harder and be more open-minded. Learning is hard. For most organizations, it is like changing a tire while driving down the road.
The ability to sense is core to moving counter-clockwise in the Cynefin model. The supply chain traditionally focuses on response, not on sensing before responding.
- Organizational Learning. Training supply chain talent is an opportunity for all. With the exit of baby boomers and early retirements through M&A, the aggregate knowledge of teams today in the supply chain is much lower than two decades ago. (Surprisingly, I find more close-minded thinking.) Using large language models enables business leaders to help employees quickly find information on their supply chains. The models are easily multilingual. The questions asked are great feedback for a business leader trying to finalize training plans.
- Listening Posts. I love mining multiple forms of unstructured data to develop a customer listening post. For example, why not mine the emails in customer service, along with the short-shipment/fulfillment data, rating and review posts, and outside-in-channel trends, to better understand the market? Mature teams develop listening posts while meeting weekly cross-functionally to understand true demand patterns better while understanding that the order does not necessarily reflect true demand.
- Use the Supply Chain as a Test-and-Learn System. Construct tests and hypotheses on market trends, sales, and supply chain portfolios using the differences in demand latency. Test and learn to understand regional trends.
- Use of R&D Insights to Close the Gap Between Quality of Conformance and Quality of Design. Think of all of the R&D notebooks locked up in some lab repository. How can we unleash the learnings through R&D testing to improve decisions? For example, I worked with a company that decided on seed supply chains. (Traits for seed inventories are determined one to years in advance based on crop testing and weather patterns.) Using ontological frameworks to match seed traits to regional weather patterns in a multiple if/then model streamlined the decision-making on which seeds to select for future characteristics in building new inventories.
- Pattern Recognition and Role-Based Alerting on Supply Chain Planning Master Data. I encourage all supply chain leaders to build a master database to understand cycle trends, lead times, conversion rates, labor costs, and on-time deliveries. Use pattern recognition and intelligent agents to push role-based alerts to business leaders based on interest.
Want More?
What do you think? If you want to explore this more, consider joining our upcoming training session on outside-in planning, focusing on applying the Art of the Possible in analytics to solve business problems. The class is limited to manufacturing, retail, and distribution business leaders. There are two virtual classes. Each class is six weeks. Each company can send two business leaders. Follow this link to access the curriculum. The class starts the last week of January.
The goal is to learn, question, network, apply, and share. Each session will have a homework activity to encourage participants to think openly. The class is supported by a large language model built in cooperation with UTHEREAL. Historically, unstructured data has trapped expert knowledge, making dissemination extremely impractical. Our goal is to open the mind like a parachute.