Waves of hype pass through supply chain narratives. Thought leaders push concepts. Tech companies rally. Without tangible results, the concepts evaporate over time. Each has a cycle, typically a large investment, but leaves no fairy dust residue.
As an old gal attending multiple conferences (more than I would like at times), I have listened to speakers waft eloquently about the value of concepts like networks, big data, industry 4.0, and digital supply chains. Did these investments drive value? I don’t think so. My 67 quantitative studies over the past decade do not support the value. Will they prove valuable in the future? I don’t know. (I could write a blog about each story, but let’s save that for coffee. I will be at CGT in Chicago, and Kinexions in Miami. I like mine black.)
A Closer Look at Digital
For a decade, I have struggled to get a clear definition on the digital supply chain. While digital marketing is clear, for me, supply chain digital management is not. For most, the implementations are focused on paperless, hands-free, and seamless transactions. Which is ok, but not transformational. I do not see companies push the envelope to define digital passports for provenance, implementing learning ontological frameworks, or schema on read data fabrics combining the graph and vector DB. For most digital became an excuse to implement more traditional relational database technologies.
This discussion takes all the oxygen out of the room yet perpetuates the belief that we are making progress. Let’s take a discussion from my DM on LinkedIn from Ryan. “Increasing digitalization means that global supply chains now have unprecedented connectivity, monitoring capabilities, and information-sharing potential. However, this increased data flow and interconnectivity also heighten operational and cybersecurity risks.” My response, “Sorry Ryan, the research does not support that increased digitalization brought unprecedented levels of connectivity. Most companies invested in enterprise transactional systems, but their networks operate primarily through spreadsheets and email. Similarly, over 95% of manufacturers invested and implemented supply chain planning, but their primary tool today is Excel. The research supports that we have not improved supply chain visibility, and we are going backwards in delivering results through supply chain planning.”
Does the Dog Hunt?
As I type this blog post, I face my flowers blooming in my backyard. They are beautiful. A pond flows quietly. Birds are gathered at the feeders. The sun is shining.
Missing from the yard are squirrels, rabbits, and other vermin. The reason? My dogs hunt. I am blessed to have three Skye Terriers. The breed innately goes to ground and flushes out vermin. Nothing excites them more than the squirrel that mistakenly makes its way into my backyard. Meet my terrier crew. They look like hairy mops, but they love a good squirrel chase.
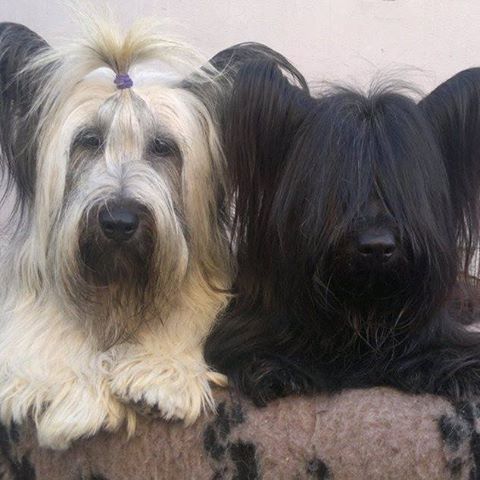
Definition: With reference to a dog used for hunting game, the colloquial American-English phrase that dog won’t hunt, and its variants, are used to express the opinion that a particular plan or approach will not succeed.
—Synonym: that cock won’t fight. Wordorigins.com
So, my question is simple. Do these new programs–hype cycles–produce results? Do these approaches (new dogs) hunt? Tech companies push self-serving agendas like a hammer hunting for a nail. Today, AI, autonomous supply chains, and probabilistic supply chain planning are in vogue. In this blog, I give my thoughts on the fit of these approaches for value. I don’t think that these dogs hunt.
So, What Who Cares? Do These Dogs Hunt?
As influencers when we write and recommend tactics, I believe that we should test and learn. I am researcher.
I grow weary of talk with hollow words. I am currently working with technology providers and business leaders to ensure that new approaches to planning help us to sidestep the roller coaster of hype. My approach is to test. I openly publish the results.
I don’t know about you, but I am tired of riding the endless wave of hype cycles. I want dogs that hunt and drive value. Here are my thoughts on the current trends.
Does Autonomous Supply Chain Planning Make Sense? I think not. Let me explain. I find the teams touting the concepts of autonomous planning are largely from IT backgrounds focusing on the automation of supply chain planning engine processing. Most are excited about their new platforms. Technology for technology’s sake, but they cannot answer the question of the “problem that they are uniquely solving.”
What I find missing in the discussions is the understanding of planning.
A successful plan requires design, collaboration, simulation, what-if analysis, and closed-loop learning. It is not about the efficiency of the batch job. I find successful teams learn more about operational potential through what-if analysis than wrote use of the first plan generated in a batch job.
The point of view of autonomous supply chain planning is often very-ERP centric. For example, today, I had an interesting discussion with a client on the dilemma of Shadow IT and 200 planners having a number of planning technologies but using visual analytics. His point was on each ERP expansion, and with every ERP upgrade, the transactional systems are upgraded, but the planning transformation seems to never happen. My question is can it ever happen with an ERP-centric view?
What is a better answer? With that said, please know that I recognize the issues of current deployments of supply chain planning. Planning teams are larger than ever, it is harder and harder to hire talent, and planners struggle to cast off spreadsheets to use deployed systems. With the growth of the global supply chain, we threw planners at the problem. Many of our planners, lack training. My suggestion is self-service by business leaders in a collaborative platform on top of traditional APS. This is the approach that I am currently testing with technology providers. The goal is to unlock the data that is currently only available to planners and make it available to business leaders. (The collaborative layer is depicted in orange in Figure 1.)
Figure 1. Outside-in Planning Taxonomy
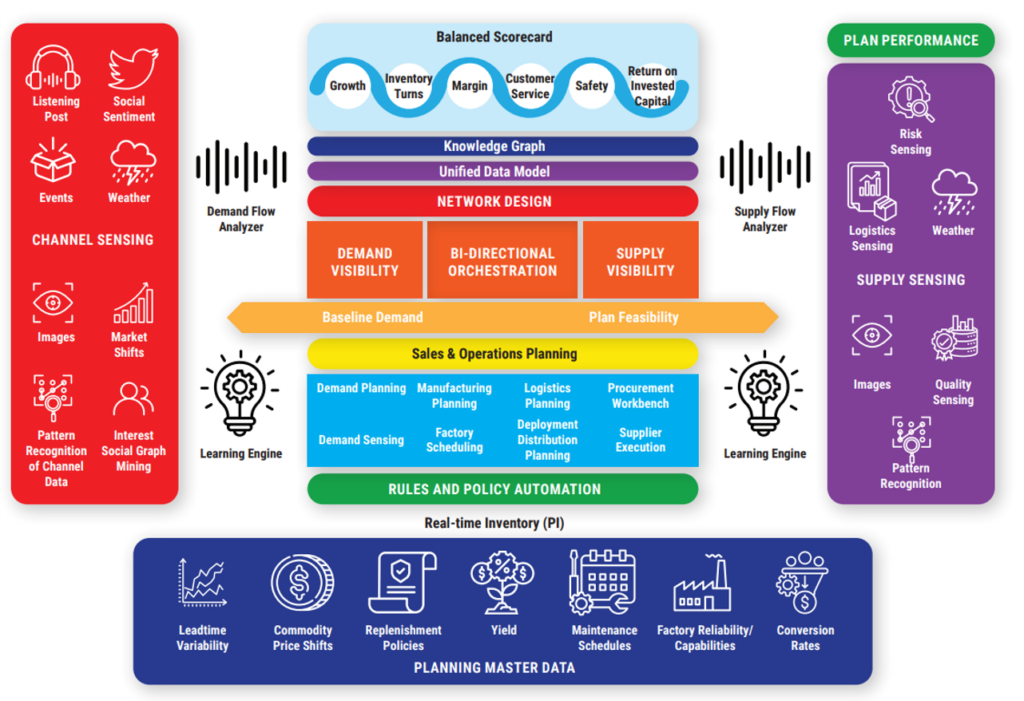
When testing planning effectiveness through Forecast Value-Added Analysis (FVA), Inventory Health, or Schedule Adherence, I find that for most clients that I work with, that their plans lack both feasibility and reliability. One of the problems is that today only planners can get to planning data. Second problem, is that few can answer the question, “What makes a good plan?”
My answer is self-service planning. I am testing the concept with a couple of technologists. Self-service planning is a collaborative layer built above traditional Advanced Planning Solutions (APS) to enable intelligent agent driven insights by role for each business leader to answer the questions of:
- How good was my plan?
- What was the plan adherence?
- What are the proactive insights from the prior planning period to take forward?
- What are the adaptive alerts that I would like to see in this planning period?
- How does the plan tie to the balanced scorecard of growth, inventory health, operating margin, customer service, and Return on Invested Capital (ROIC)? What do we learn through gap analysis?
Future for Probabilistic Planning? Probabilistic planning is a type of engine to drive planning insights. Conventional technologies are deterministic (single number) while probabilistic engines show the results in a mathematical distribution (think statistics class). The engine then uses the statistical pattern of all of the data to translate demand into a safety stock value. This technique has been very useful for retail store inventory and MRO where demand is lumpy, latent, and difficult to forecast. Makes sense. Right?
My question then centers on how do we drive a probabilistic signal through manufacturing? How do we translate the statistical pattern into planned orders in tactical planning and manage constraints. And what is the role of the combination of probabilistic planning and the bullwhip effect on the right decision for sourcing? (The current taxonomy does not include a tactical planning tool for procurement (above MRP) which is an opportunity.) How should procurement execute forward buys, give visibility on procurement constraints, or drive bi-directional orchestration across make, source, and deliver? I do not see the testing and definition of engines for this type of decision support. So, my conclusion? The handwaving for probabilistic planning is mainly about safety stock which represents only about 15% of total inventory for the Global Fortune Manufacturer greater than $15B. So, does this dog hunt? Sometimes. Is it a hunter for all seasons and occasions? There is much more work to do. When companies speak to you about probabilistic planning ask them to draw a taxonomy and show how constrained manufacturing, supply, and transportation signals are consumed into a probabilistic model. Ask them to draw the flows for bi-directional orchestration considering the trade-offs of make, source, and deliver. I cannot find an answer yet.
In addition, probabilistic models are not a fit for all types of demand flows. Even on a lumpy and enterprise flow using a probabilistic model with experienced modelers, I see only a 2-3% MPE improvement. However, the use of probabilistic modeling using market data shows a 5-7% MPE improvement. But let’s don’t forget the consideration of adding complexity in an already complex environment where many don’t understand planning. The result? You may have an expensive dog, that is an occasional hunter.
What are the Use Cases for Artificial Intelligence? I shudder when I see technologists plastering all of their marketing with AI. Your question should be, “AI what? And, why?” Ask the technologist to define Artificial Intelligence (AI).
I am excited about the use of large language models (Generative AI) for R&D learning. (Close your eyes and think about all of the lab books, computer records and testing by R&D.) The goal is to drive benefits to better define quality of conformance. I am currently speaking to a seed manufacturer about the building of a large language model to define seed traits, and to select the seeds to inventory for next year’s crop, based on current crop conditions. I am also excited by the use of intelligent agents to feed improved insights on product availability based on insights from a planning master data layer (reference Figure 1) and across networks.
Narrow AI works for me a type of engine to improve planning, but the discussions on engines must also include the definition of the right model. Based on the current issues with the distortion of history from the pandemic and changing market conditions, companies need to constantly evolve both models and engines. Attribute-based models, or the redefinition of a demand planning hierarchy for better bottoms-up and tops down forecasting, may be necessary. Most companies’ deployments of item-based forecasting based on a ship from model is limiting.
So, there are many forms of AI. Ask the technologist to be specific in the use case.
And, then there is a discussion of data. Based on testing for three years, I find a market-driven model using market data beats any deployment of combinations of enterprise models/engines. Despite the choice of engine, start with the definition of demand flows and the use of market data. The issue is demand latency with the long tail of the product portfolio introducing bullwhip and distortion.
Wrap-up
Next week, my LinkedIn feed will be full of the hollow words of anti-fragile supply chains using probabilistic planning in an AI environment as a step to autonomous planning. If you are in Barcelona, raise your hand, and ask, “Can this dog hunt?” Are there tested use cases? Are we clear on the problems we are solving? Or is it more hype in the social media word salad spinner confusing the audience and delaying the achievement of true value?
My opinion is that you should put your hands in the air like you are on a roller coaster. The word salad spinner will take you through many ups and downs and scary turns. Don’t close your eyes but get ready.
I prefer dogs that hunt. I hope you do as well.
Based on the current hype cycle, I am sure that this blog post will generate a lot of dialogue. I feel the more discussion the better. Bring it on.
For additional insight check out other content: