Earlier this week, I started a blog series on Big Data Supply Chains. This is the second blog post in the series.
In my prior post, I argued that if we are going to build effective architectures from the customer’s customer to the supplier’s supplier that we need to embrace the concepts of Big Data Supply Chains. This includes using new types of data and exploiting the increasing power of computing.
I also believe that we need to up the ante and change the game. What do I mean? With these advancements in computing, we have a new opportunity to redefine the output, the goal and the cycle of supply chain technologies. With these advancements, I also think that we have the opportunity to change the plumbing. The 1990s definition of integration is obsolete.
I believe that an architecture that combines Enterprise Resource Planning (ERP), Advanced Planning Solutions (APS), Supply Chain Execution (SCE) Systems plus Business Intelligence (BI) is not sufficient. Why? Today, supply chain architectures respond. In most cases, it is not even an intelligent response. In fact, it is a DUMB, SLOW and often INACCURATE response. Current technologies either help us make better decisions through the use of optimization in planning or through improved visibility of enterprise transactions.
The data is dirty. The latency of information is long. Most companies have invested in enterprise technologies on a project basis. For most users, satisfaction is low. It should be no suprise that Excel is the number one planning application.
Today’s technologies are primarily about supply. Deep solutions for demand are needed and an untapped opportunity. I believe that the future of supply chain technologies will define processes from the outside-in based on a deep and comprehensive solution for demand. Solutions that sense, shape and drive a profitable response bidirectionally from sell-side to buy-side markets.
If used correctly, I believe that the emerging technologies can allow us to drive a more intelligent response than we were able to achieve in the 1990s through optimization alone. I believe that through the concepts of Big Data Supply Chains that we can evoke the power of computing power to help our supply chain networks not just respond, but to dynamically sense, listen and learn. And, for the more advanced companies, I believe that they will fine tune their architectures to sense, listen, test, shape and drive continous learning. It is the dawning of a more agile supply chain platform. Machine to machine learning can help our supply chains continously learn.
New approaches are emerging, if we can be open to the outcome. It is a time to learn, unlearn and relearn. The other day, I was interviewing a VP of Supply Chain about the future of supply chain technologies. I asked him, “If he had a magic wand, how would he describe what supply chain technologies of the future would look like?” His response, “Lora, I don’t know. I am frustrated. I just know that what we have does not work very well. Somehow, we need to be able to have a more agile sensing platform. Our current architectures are too rigid and the response is too late.” For reference, he works at a global company that is very advanced in supply chain thinking. They have 19 instances of SAP for ERP, and have gone through five different solutions of Advanced Planning (APS), and have superalitive systems for order management, warehouse management, and transportation management. They were also early adopters of Multi-tier Inventory Optimization and Strategic Modeling technologies.
If you buy my argument, it is time to retool and learn a new jargon. There is a powerful opportunity for line of business leaders to lead and define the Art of the Possible for Big Data Supply Chains within their organizations. Here are new terms to know:
Big Data Supply Chains. Each person that you talk to will define this differently. When it is used in a business concept, ask what the user means. There is no standard definition, but in general, it means a dataset that is too large and awkward to use conventional relational data base techniques for capture, storage, search, visualization and sharing of data. It is the world of terabytes, exabytes and zettabytes of data.
Columnar Store. A type of database management system that stores information by column versus by row. Columnar databases enable in-memory processing, column pruning and compression. They enable outrageous compression factors, it is not uncommon to compress a Terabyte of traditional row-store data into tens of Gigabytes. The advantage is the ability to aggregate similar data to increase computational speed. SAP HANA architecture is an example of advances being made in in-memory processing through advances in columnar store architectures. It has advantages and disadvantages. I believe that SAP HANA will help us with visualization of large data sets, but it is far from a panacea to help redefine supply chain architectures. IBM, too, provides columnar database capability to speed data warehouse queries. The IBM Smart Analytics Optimizer provides this capability withDB2 relational DBMS on z/OS (mainframes), and related technology like the Informix data warehouses (e.g. the Informix Warehouse Accelerator).
Fuzzy Logic. A form of computer reasoning that is approximate versus binary logic that is fixed and exact. It enables decision making that is not “black and white” where the best answer lies in understanding the range between completely true and completely false. While optimization helped drive business intelligence in the 1990s, new forms of pattern matching and the use of fuzzy logic will be combined with artificial intelligence to drive new ways to sense, act and then respond. For an early solution in this area, check out Enterra Solutions.
Hadoop. A framework designed to support data-intensive distributed applications to support thousands of nodes and petabytes of data. It is often referred to as open source Apache Hadoop and is being designed by global community using Java. Yahoo is the largest contributor. It is new and largely unproven for use by product manufacturers. IBM builds on Apache Hadoop with its InfoSphere BigInsights product to provide an analytic infrastructure for massively distributed data.
MapReduce. MapReduce is the framework of Hadoop. Introduced to the market by Google in 2004, this software framework uses map and reduce functions commonly used in functional programming to speed processing through distributed computing on large data sets on clusters of computers. There are few use cases for the supply chain, but Teradata’s acquisition of Aster Data opens up new possibilities to combine MapReduce and SQL to solve big data supply chain problems. It makes the processing of distributed semi-structured data easier.
Pattern Recognition. Pattern recognition uses fuzzy logic to recognize sets of data like others and identify patterns in large data sets.
“R” A freeware or open source programming language for statistical computing and graphics. Recently, it has been widely adopted by statisticians for developing statistical software and data analysis. R is not well suited for big data problems unless you like to write tons of code. It has been widely adopted by bio-informatics but has yet to penetrate the larger analytics market. Companies will be constrained by architectural memory limitations of R, but the open source nature of R will enable data-centric processes.
Natural Language Processing. To harness the power of unstructured, electronic text data in machine learning.
Ontology. A rules-based approach for semantic association and category relations. We are seeing the use of rule-based ontologies in the evolution of Sentiment data (SAS), Supply Chain Execution (Enterra Solutions) and Supply Chain Risk Management (Dunn and Bradstreet/Open Ratings).
Semi-structured data. A form of data which contains both structured and unstructured components. It does not conform to formal structural definitions of relational data base tables and data models, but can may contain some defined fields, such as subject line or date, in addition to free format text data, such as the body of an email.
Unstructured data: A data set without pre-set structure. Unstructured data abounds in call-center logs, social listening, contract, servicing and warranty data and risk management applications. Early applications to harness the power of unstructured data for the supply chain is Dunn and Bradstreet’s application Open Ratings and SAS Inc.’s Social Media Analytics application for social media listening.
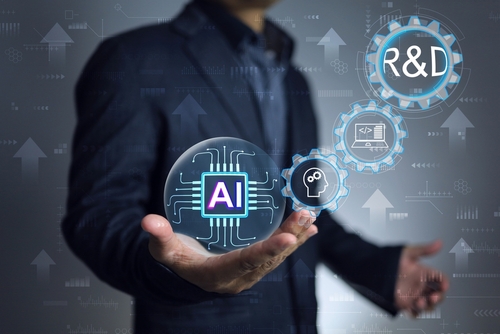
My Favorite AI Use Cases
Traditional supply chain planning was defined by the theory of constraints and the Deming Wheel of Plan, Do, Check, and Act (PDCA) philosophies. The PDCA