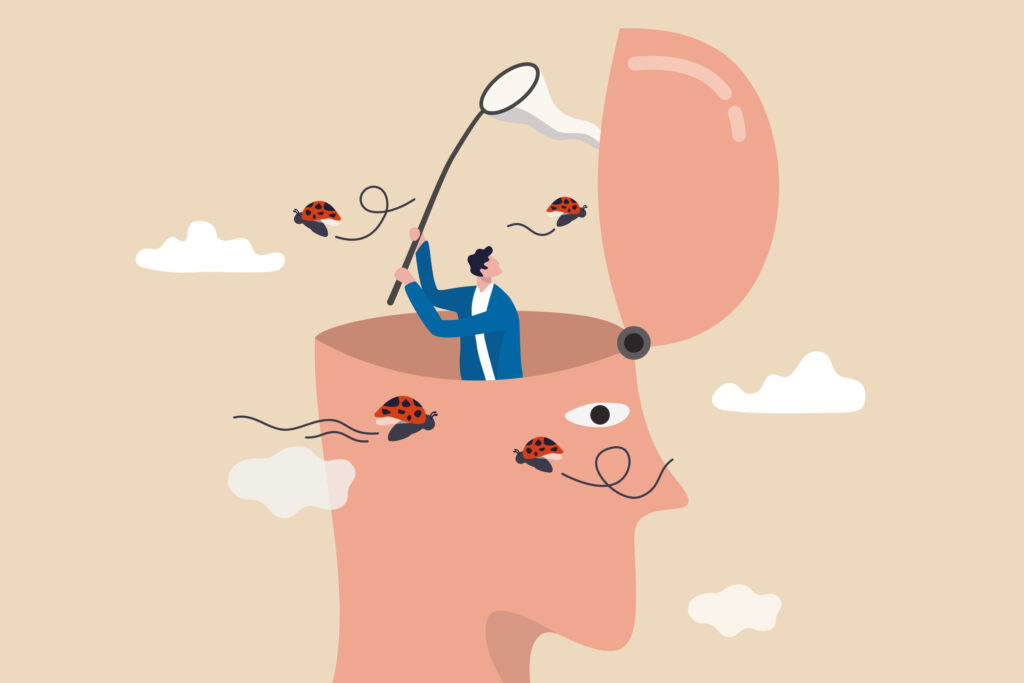
Supply chain leaders readily agree that industry differences exist in planning. Similarly, debate rages on the differences between regions. Industry differences trump regional definitions, but there is little recognition of the supply chain planning requirements by company size in the discussions. When it comes to defining solution success, mapping the requirements based on company size is more important than industry or region.
Today’s planning solutions serve small regional teams well, but the answer for the global multinational manufacturer requires bespoke wiring of solutions from multiple technology solutions. There is no good answer.
Figure 1. Analysis of Quantitative Surveys From the Last Decade
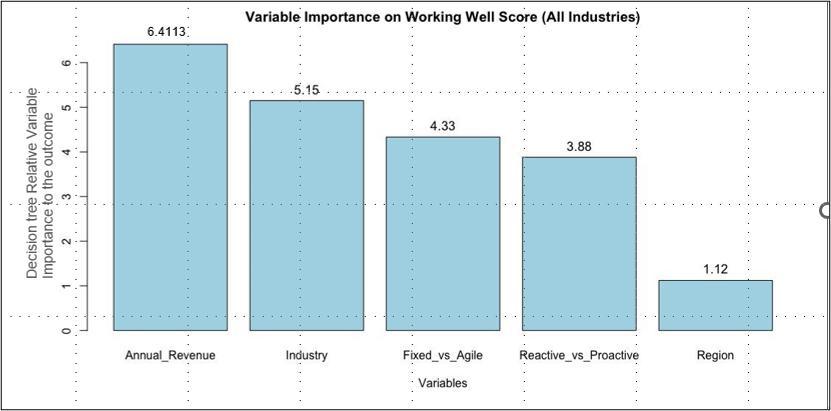
Getting To An Answer
The first step is to recognize the gap. In my interviews with global multinationals, I hear the need for an architecture that scales for hundreds and thousands of users. There is a need for discovery and what-if analysis, enabling insights not held hostage by laborious, time-consuming processes to run multiple batch jobs. Companies have not been successful in building planning solutions themselves. At the same time, academics focus on building better mousetraps (improving models and engines) in existing taxonomies versus redesigning work to ensure the security of the system of record and proactivity in systems of insights.
Figure 2. Rethinking Planning
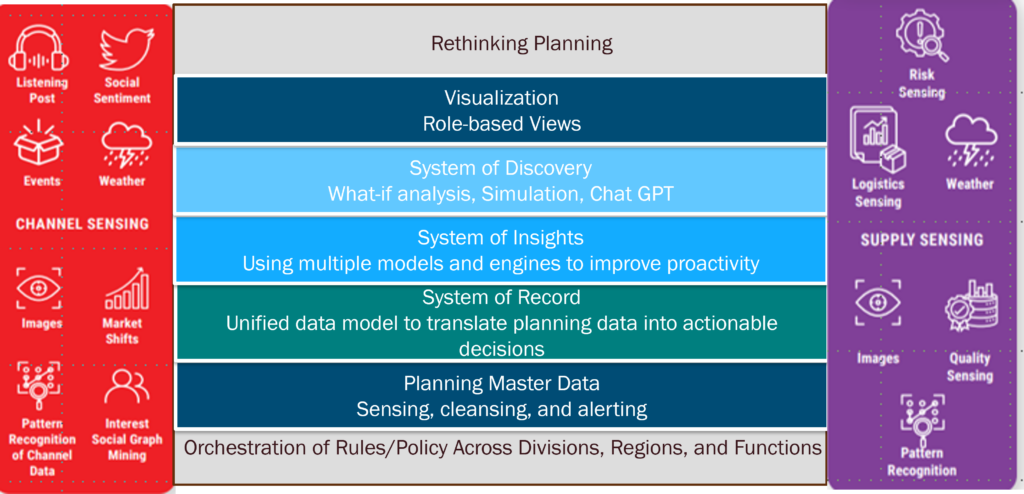
As we think about the promise of new forms of data analytics, the talk of “AI” without definition drives me crazy. There are so many techniques under the “AI” umbrella. The tragedy, I find, is when technologists slap the term “AI” on their marketing without sharing the specifics of how specific tactics drive outcomes. AI for AI sakes drives nothing other than the hype cycle. Here are some application possibilities:
Architectural Element | Technique |
Planning Master Data | Pattern Recognition and Machine Learning |
Channel Sensing | Large language models, Sentiment mining, Machine learning, and Graphical flow modeling |
Supply Sensing | Large language models, Retrieval Augmented Generation (RAG), Vector DB, Machine Learning, Pattern Recognition |
Rules and Policy | Rules-based Ontological Frameworks |
System of Record | NoSQL No/Low Code, Unified Data Model |
System of Insights | Agent-based Optimization, Probabilistic Demand Translation, Cognitive Learning Models |
Systems of Discovery | Digital Twin Simulation and Optimization |
Visualization | Workflow Automation and Role-based Views |
Wrap-up
I look forward to hearing your thoughts. Why do you think we missed the mark in serving large, global multinational corporations?
And how do we stop the false AI rhetoric that has the industry spinning its wheels instead of addressing possibilities?