In 2012, I published an article on Sales and Operations Technology (S&OP) Maturity. (I never republished the report, because not enough has changed to warrant it.) The report centers on the concept of moving from inside-out to outside-in technologies. At the time, one of the people (let’s call him Noel) that I great respect for in the industry, called me to say, “Lora, I don’t get it. Why is there a discontinuous line on the model?”
Noel is one of the 84 sign-ups in my outside-in training (three virtual classes starting next week), and I hope that he has a better understanding at the end of the class. Here I write on what I hope that he learns.
Understanding the Model
This model reminds me of a snail. In each phase, companies refine the models until they find that the future is discontinuous. To use channel data, they must redefine and connect commercial models, and to use supply data, they most redefine transportation and procurement processes. (Companies slowly learn that to successfully use network data to sense and respond bidirectionally, you must redefine the enterprise model to be outside-in.)
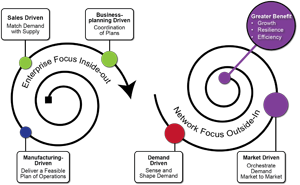
The evolution of planning moves from a focus on the enterprise to an adaptive platform that senses and responds based on market data. The enterprise-centric models, due to the lack of adaptability, cannot shift to use market data. This is not a lift and shift proposition. An outside-in model requires a redefinition making today’s supply chain planning technologies legacy investments.
The growth of Artificial Intelligence (AI), unstructured data mining and pattern recognition, along with NoSQL enables this redefinition. In the evolution, companies find that 80% of data surrounding the enterprise’s supply chain is unused in current supply chain decisions. Most companies do not use all the data available. The reason? The data is in organizational pockets. A second misconception is that the data must be a complete data set or clean. With narrow AI and pattern recognition breakthroughs, this is no longer the case.
What Is an Inside-Out Planning Process?
An inside-out planning process is the current state. The models use enterprise data and the outputs from the models are pushed to trading partners. The problem is two-fold: latency and political bias. An inside-out model, based on testing, is weeks, and often months, out-of-step with what is happening in the market. (For the purposes of this blog, market data is channel, logistics, and supplier data generated outside the organization.)
The primary inputs into an inside-out planning model are shipments or orders for demand and Enterprise Resource Planning (ERP) inputs for supply.
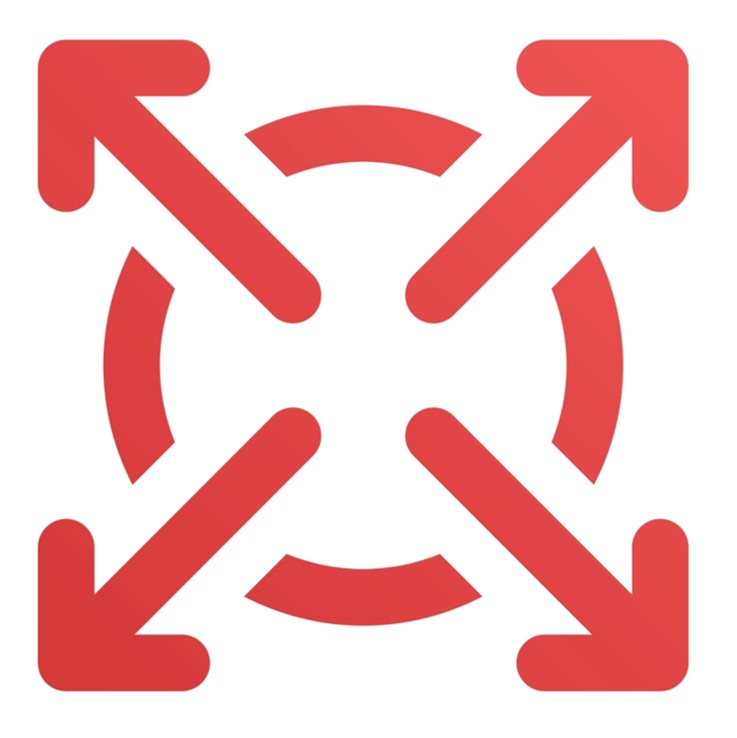
What is an Outside-in Planning Process?
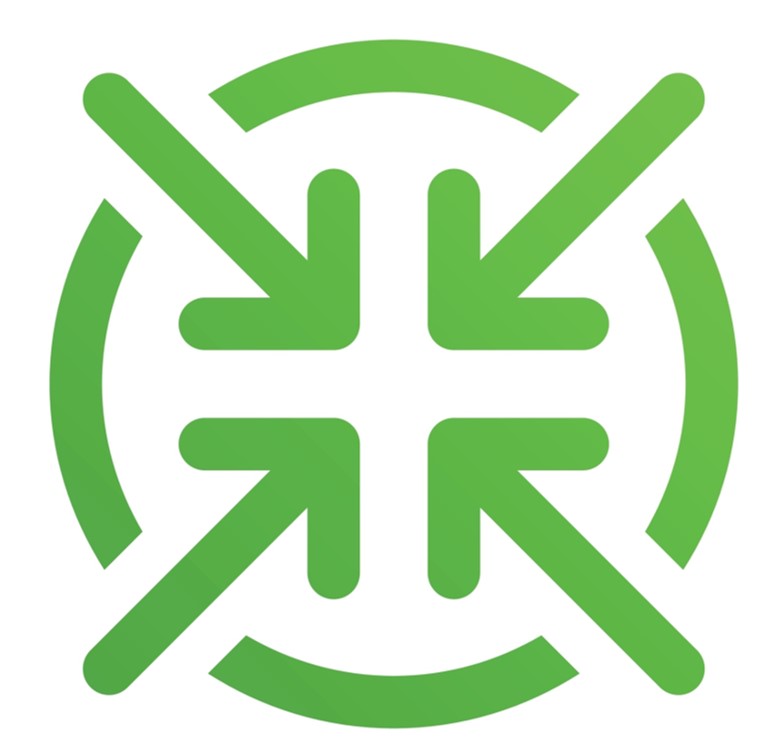
In contrast, in outside-in planning, the models primarily use market data for inputs. This includes channel data (point of sale, distributor data, warehouse withdrawals), effectiveness of demand shaping programs (trade promotions, price, marketing programs, events, and new product launch) along with supplier data (constraints, quality data, on-time delivery, schedule adherence and ESG impact) and logistics information (constraints, delivery performance, cost, trailer temperatures, and ESG impact). In the outside-in planning process, the data model output is refined and published to a series of networks. The model recognizes that relationships are not linear and focuses on synchronization and harmonization with the goal to improve interoperability between trading partners. The company readily seeks to improve baseline demand while analyzing the impact of demand shifting versus shaping. (When a demand shaping activity fails, product is shifted from period-to-period. This increases cost but does not improve baseline demand.)
Why Does It Matter?
One of the issues with an inside-out signal is the use of the order (or shipment) as a proxy for demand. This assumption has five issues as explained in Table 1.
Table 1. Issues with Using the Order as the Proxy for Demand
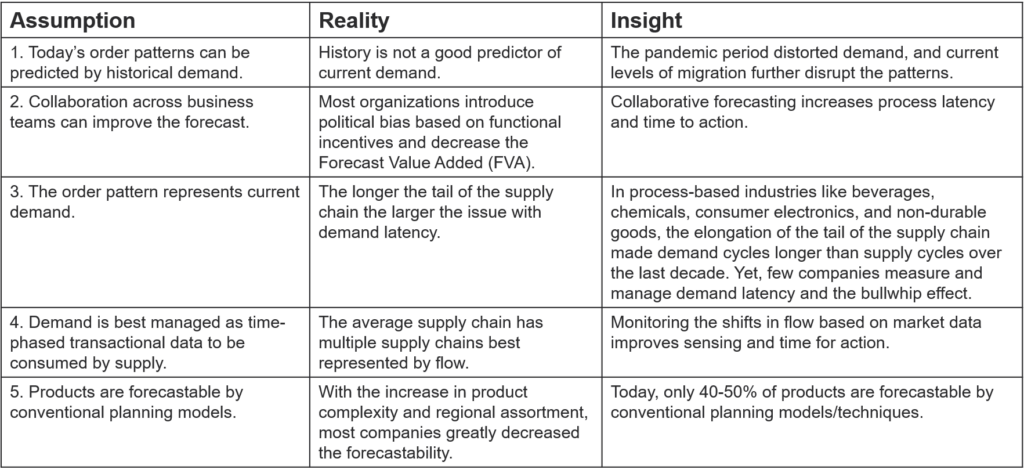
The term demand or order latency is new for some, so let me explain. The order latency is the time from purchase by the end consumer to the visibility of the order by the manufacturer. The higher the volume and the more forecastable the product, the shorter the demand latency (usually days within two weeks).
Definition. Demand or order latency accounts for the process of multi-tier replenishment. For example, when a product at retail is purchased, the shelf is replenished from backroom stock. With the use of backroom stock, a threshold is reached to order from the retailer’s warehouse. When the minimal order quantity is reached, the retailer places an order to the retail. The time it takes to translate channel purchase to an order is demand or order latency. The longer the tail of the supply chain product portfolio, the greater the demand latency.
With high volume and predictable products, this cycle is days and weeks. However, with the elongation of the tail as shown in Figure 1, the demand latency becomes months. Over the last decade, product proliferation and the long tail increased demand cycles to exceed supply, but few companies understand the concepts of demand latency.
Last year, I worked with an allergen manufacturer that had a seasonal product with order latency of 182 days. As a result, the use of market data (air quality, pollen types, and weather) was essential to hit a tight window with the right product.
Figure 1. Demand Latency and the Long Tail
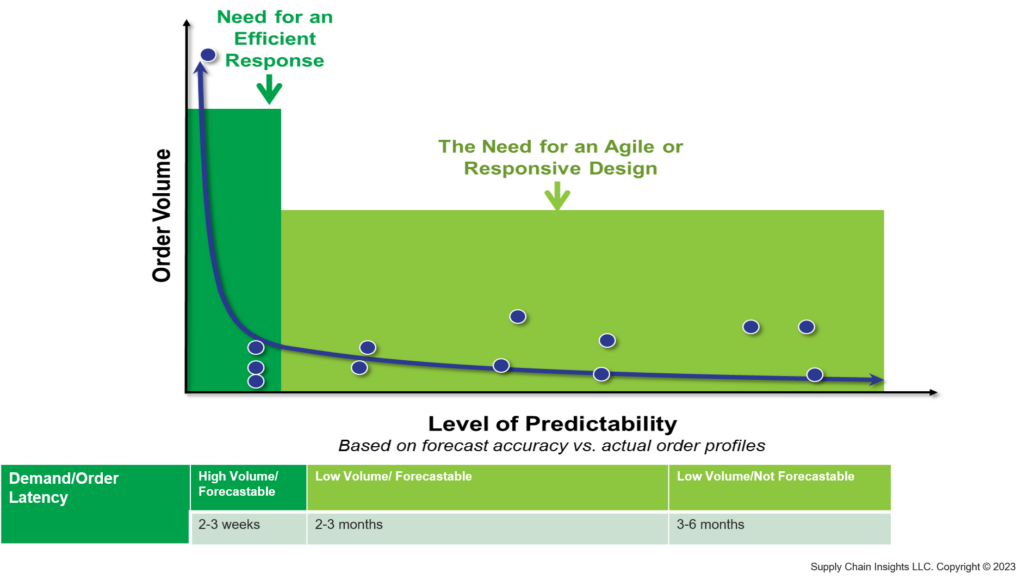
Another common misconception is an outside-in process is only about demand. Nothing could be further from the truth. Outside-in processes orchestrate demand and align supply based on pre-determined levers. As shown in Table 2, this is very different than the concept of concurrent planning.
Let’s start with a definition of concurrency. I find that it is a frequently used term with many different definitions. As shown in Table 2, there are three commonly used definitions.
Table 2. Differences Between Concurrent Optimization and Bi-directional Orchestration
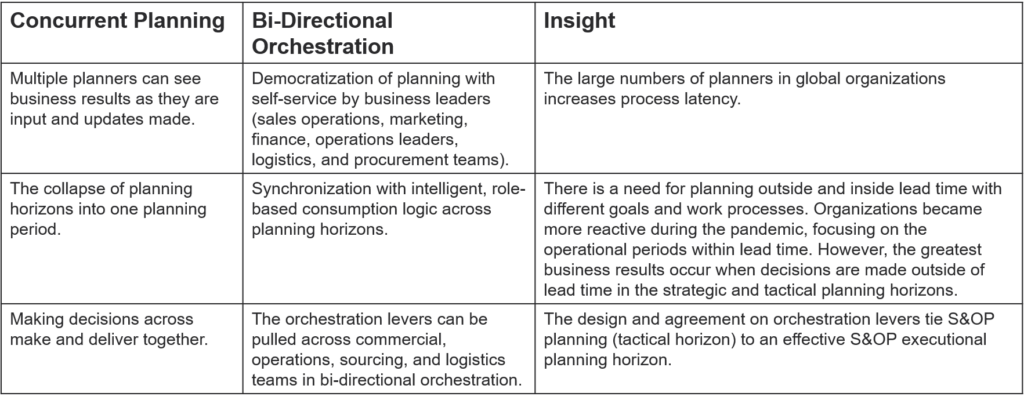
And just to be sure we are aligned, here are the definitions of the time horizons. Clear definitions of what drives a good decision in each time horizon, along with clarity of governance is essential for planning.
Tactical planning focuses on decisions outside of lead time whereas, the operational planning horizon, focuses within lead time (typically 14-16 weeks).
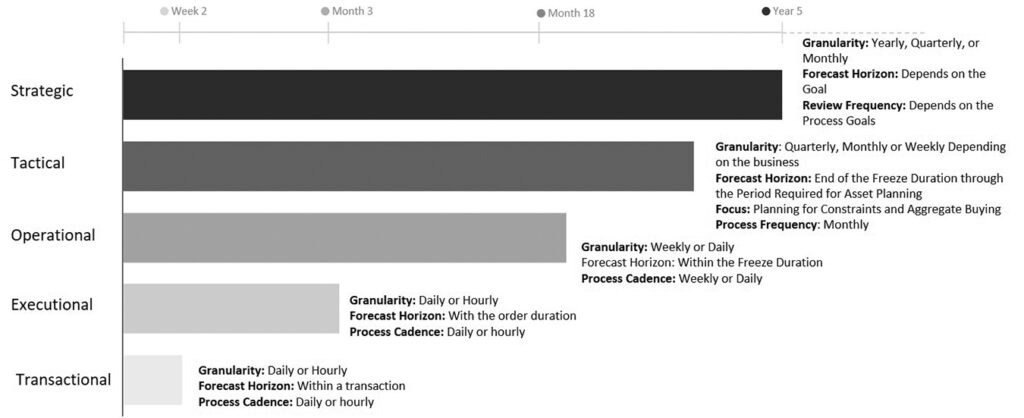
This shift in architecture for planning enables bi-directional orchestration from market (channel) to supplier (supply network). This is a natural fit for narrow AI and new forms of analytics. Note that to drive orchestration, we need to align on a balanced scorecard and build a unified data model across disparate planning solutions that exist today.
Figure 3. Bi-Directional Orchestration
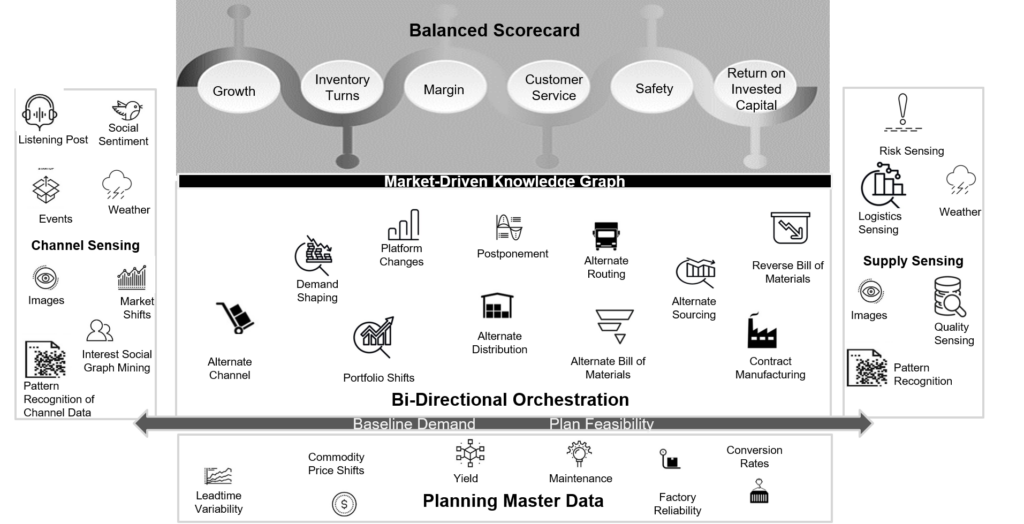
So, why does it matter? If an organization is going to make decisions at the speed of business with agility and alignment, there is a need to redefine planning outside-in. Reducing demand cycles reduces the time to make decisions. To do this though, we must learn about the current planning processes to unlearn to rethink planning. In this journey, I find that the technologists and consultants struggle the most.
Conclusion
Noel will be in my class. I will be judging my ability to teach based on his ability to see the difference between inside-out and outside-in planning models. The key learning is that you cannot just put new forms of data into an outdated model. Think he will get it? I hope so.
What do you think of the outside-in planning concepts? I look forward to hearing your feedback.
Update and A Favor
What is the value of a network? There is no definitive answer. I am trying to understand the benefits of networking synchronization in contract manufacturing.
If you enjoy the content we share, and read our blog, I am hoping that you will help. Our philosophy is that we give to you when you give to us. The primary content on the blog comes from surveys filled out by the readers. To this end, I welcome your participation. (We always share the data anonymously.) If you fill out the survey, we will share the data to use with your management group.