Drip. Drip. Drip.
Industry 4.0. Drip
Drip. Drip. Drip.
Big Data. Drip.
The Connected Supply Chain. Drip
Digital Supply Chain. Drip.
Autonomous Supply Chain Planning. Drip.
Self-Healing Supply Chains. Drip.
Touchless Supply Chains. Drip.
Many messages to the market, but where is the value? I have developed an allergy for word speak and hollow promises.
Drip. Drip. Drip.
Background
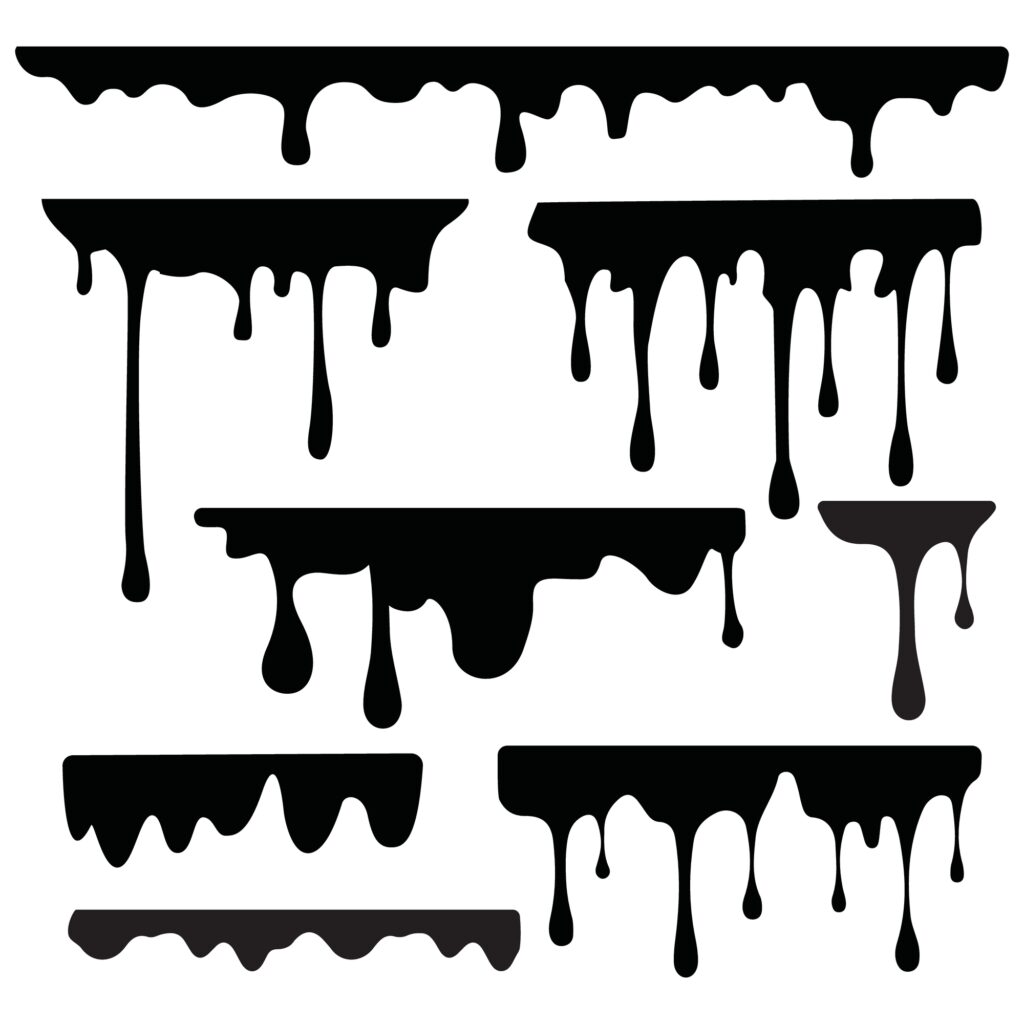
I find that each conference provides the audience with a new framework to consider. The focus is on unveiling a new, bright, and shiny object. But, as the dust settles, is there value? And what is value? For my long-time readers, you know that fewer than 3% of companies outperform their peer group in our Supply Chains to Admire analysis, and that the Gartner Top 25 is essentially a beauty contest for underperformers. The companies that people believe are top performers typically are laggards. Small companies outperform large companies, and the marquee customers of major supply chain planning technology providers underperform. The issue is that when companies optimize functional metrics, they throw the supply chain out of balance and sub-optimize value. The most efficient supply chain (in terms of lowest cost) is not the most effective.
Supply chain leaders love bright and shiny objects. Today, the bright and shiny object is AI. But what is AI really? And is there value for planning processes?
Artificial Intelligence comes in various forms, including generative AI (large language models), narrow AI (an optimization technique), machine learning (for pattern recognition), and agentic AI (intelligent agents). Last year, generative AI was the hot topic, while this year, the focus is on agentic AI. All our great tools in our toolbox to improve supply chain planning, but my observation is that we are trying to AI stupid. Let me explain.
Fundamental Shifts.
We are finally starting to see a shift from schema-on-write planning taxonomies to schema-on-read. Why is this important? Traditional approaches built optimization on top of relational databases. To use optimization, the data had to be cleaned and stored in pristine condition in a data jail (rows and columns of traditional database technologies). There were several problems— the technique was only applicable to structured data (most of the data surrounding the supply chain is unstructured), and the data is constantly changing. The approach also stripped the semantic layer, limiting usage.
The good news is that in the last year, Kinaxis, OMP, and O9 have fundamentally changed their architectures to use semi-structured data. Kinaxis even recently announced a partnership with Databricks. This shift improves modeling options and the use of disparate data. The problem is that the industry is applying new techniques to the existing planning taxonomy without embracing the opportunity. Which makes me ask, why AI Stupid? The discussions make me itch.
Consider several factors rooted deeply into change management:
The Role of the Planner. Planners evolved in the 1980s when planning systems were not scalable and memory was expensive. In the early days of planning, we had one or two planners who cleaned the data and interpreted the outputs. Today, organizations have hundreds and thousands of planners. I liken it to the typing pool of the 1980s. Redefining the role of the planner is a prerequisite to harnessing the value of the new approaches. The reasons are many. Business leaders are unable to access planning data and model outcomes. We have not designed the planning systems to serve managers, directors, and vice presidents, aiming to improve decision-making and collaboration across the source, make, and deliver processes. There is no unifying data model to align procurement and manufacturing, transportation and distribution, revenue management and demand planning, or sales account teams with supply chain planning. This could all change if we discard our current definitions of supply chain planning and start anew. We should not rely on AI to be stupid; the goal should not be to make the planner more efficient. Instead, I believe that the goal should be to help the organization make better decisions. The role of the planner needs to change, but this shift cannot happen from the inside-out.
Redefine Your Organization’s Relationship with Data. In this new world, data does not need to be perfect, and the list of new processes and capabilities to use unstructured data is endless. The starting point is to redefine your relationship with data. The next step is to throw away the conventional definition of supply chain planning. Companies need a planning system of record, but the models become multiple inputs to multiple outputs that are ever changing based on learning. While convention focuses on optimizers and engines, in this new world, the focus is on model building based on what drives value. Rules engines, learning models, and agentic AI need to be built around what drives value. Automation of traditional planning taxonomies focused on cost reduction sub-optimizes market capitalization/employee. The third step is to do a data inventory. Explore the data the organization owns and explore how to use different forms of data to answer the questions central to the business.
3. Focus Outside-in and Orchestrate Market-to-Market. Using channel and trading partner signals build outside-in models and orchestrate decisions across source, make and deliver.
In short, don’t AI stupid. Rethink planning based on the art of the possible and drive value.
So, What Is Value?
When I studied at Temple for my DBA, I did an academic search on what is value in the supply chain. There is no answer in academic literature. Over the last two years, I have been involved in a structured program with Georgia Tech. Using the objective function of market capitalization/employee, we find that 40-70% of value can be modeled using the four metrics of growth, Return on Capital Employed (ROCE), inventory turns, and operating margin.
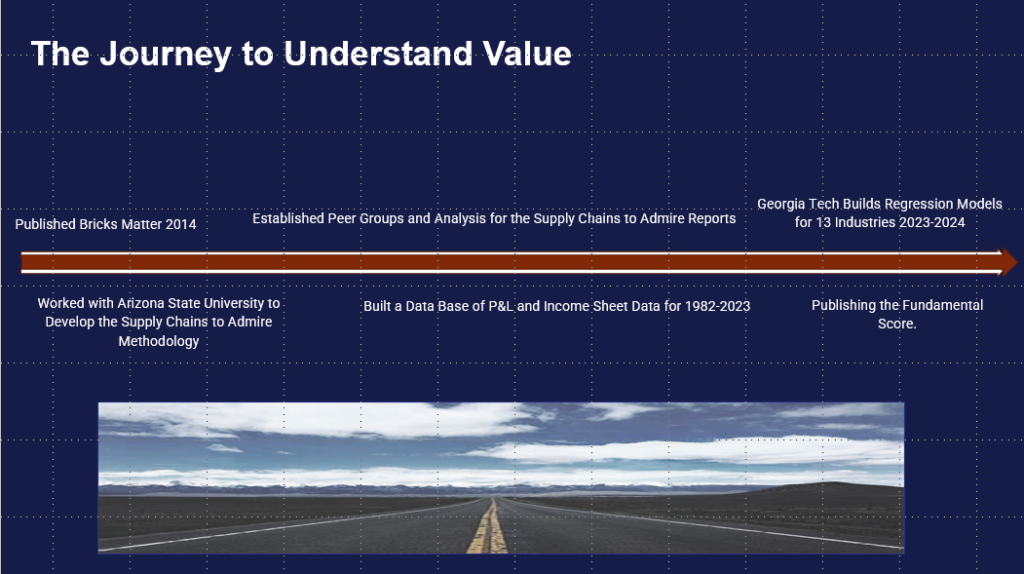
This month, we will release the Supply Chain Fundamental Score. Use this methodology to engage your organization on what matters and what defines value. But please don’t put AI into traditional supply chain planning processes because if you do your organization will experience the drip, drip, drip of declining value.