“We have too many planners. And even if we can afford the costs, we don’t get good answers with our current forecasting processes,” stated the supply chain leader on the phone. “I want to build outside-in processes, but I don’t know what this looks like. Can you tell me what you mean by this statement?” My question to her was “Do you have the courage to upend the apple cart?” The essence of this discussion is shared in this post.
In the 1980s and 1990s, when supply chains were simpler and more regional, supply chain planning technologists developed software to apply optimization to shipment and order history to predict the future. (At this time, history was more predictive of the future.) As supply chain processes matured and demand latency increased, the order became less representative of demand. Why? The addition of products at the item master, and the focus on a localized and personalized response, increased demand error; but worse, it made the demand signal lumpier and less predictable. Demand latency–the translation of channel sales through reorder points to produce an order–has increased 50-70%. Yet, we continue to push traditional practices as best practices and we are unwilling to rethink demand processes.
To Fix the Problem, Question Current Thinking. Inherently, business leaders know that traditional forecasting processes are inadequate. Cognitive computing/machine learning can redefine decision support/forecasting processes. The shift in analytics capabilities opens new doors. Early adopters are starting these tests. As shown in Figure 1, forecasting is the largest gap; yet, we continue historic practices, pouring more and more manpower against the problem. Let’s face it, the 1990’s approaches are inadequate.
Figure 1. Gaps in the Five Year Views
Channel Data and Point of Sale Data is Essential and Necessary to Reduce Demand Latency. The concepts of demand latency are not well understood by business leaders. With the focus on growth, new product launch and forecasting special items increases in importance. The demand latency of new products and special items is high: often 10-20X that of regular turn items. Without the use of point-of-sale data, companies cannot sense market shifts quickly enough to respond and drive effective replenishment of these items critical to fuel growth. As a result, companies are always on the back foot. As shown in Figure 2, the prevailing view of the value of point-of-sale data is strongly held by technologists, but not by line-of-business users. The technology providers have not been able to prove the value of outside-in processes based on point-of-sale data.
Syndicated data, by definition, is less accurate and has more latency (usually a 2-3 week lag to the market). Yet, the cost to get syndicated data in consumer value chains is so high, that it is difficult to get funding to collect, synchronize, and harmonize point of sale data.
Figure 2. Differences of Beliefs in Types of Data
Gaps in Revenue Management. The relationship between demand shaping–price shifts, marketing/advertisements, promotions/rebates–and revenue management is not well-understood. While business users feel that current applications are adequate, the technology providers developing the solutions have a different view. They see them as ineffective. Unfortunately, the technology message is usually about the technology, not the value proposition, so the market continues to dance in a stalemate.
Unfortunately, while business leaders know there is a problem, there is an unwillingness to act. Today’s solutions are not adequate. Yet, few will test an alternative approach. The good news is that new solutions offer promise. The bad news is unlearning traditional forecasting processes is hard. Business users need to be open to testing new approaches and technology solution providers need to prove the value.
Likewise, technology providers need to stop touting vendor-speak and prove the impact of outside-in processes. The use of cognitive computing on point-of-sale data is a great place to start.
We must have the courage to admit what we have today is not working, and build/define a new approach.
What do you think? I welcome your thoughts.
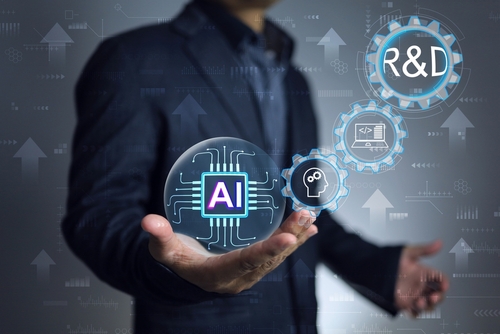
My Favorite AI Use Cases
Traditional supply chain planning was defined by the theory of constraints and the Deming Wheel of Plan, Do, Check, and Act (PDCA) philosophies. The PDCA