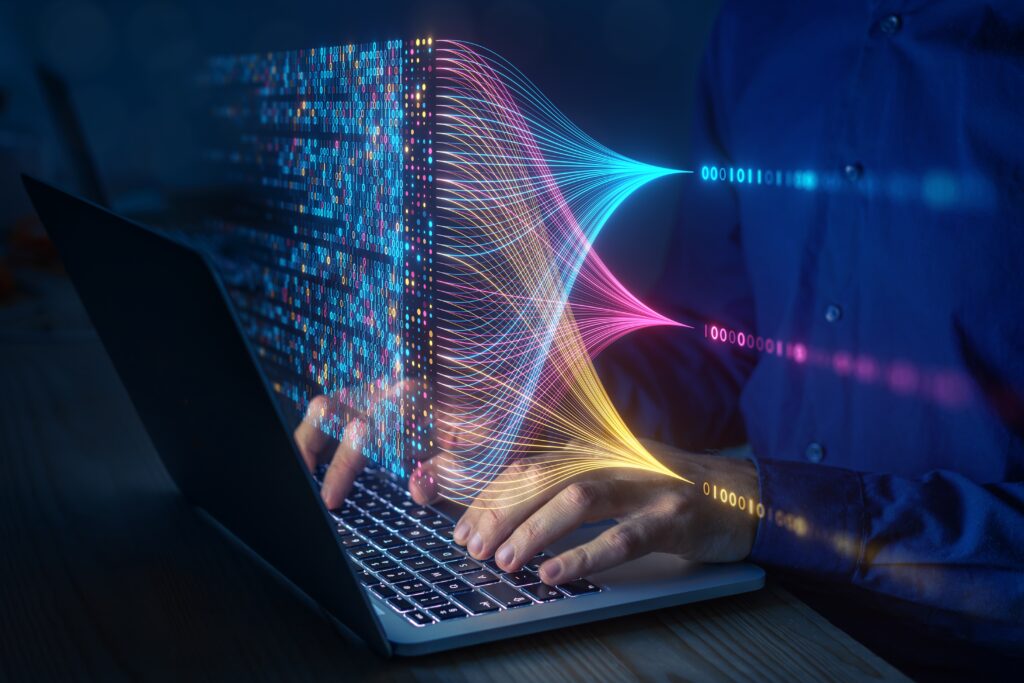
Holding my coffee tight, I gingerly slid into the last seat on the bus. It was early morning following a long night of travel.
I courteously introduced myself to the stranger sharing my seat. My new seatmate begrudgingly answered back as we often do at a conference. As we bounced down the road on what seemed like an endless journey, I asked him about his job. He shared that he worked for a freight forwarder providing supply chain planning services for a major retailer.
His company had just implemented SAP IBP, and he was not a fan. I asked if he had tested the models by backcasting. He shrugged his shoulders. The organization had little energy to test forecasting models. As a result, the project implemented by KPMG was primarily a technology implementation. The software was never tested.
I then asked about his success with Forecast Value Added (FVA) analysis. Again, I got a shoulder shrug. As he spoke, it was clear that the goal was to complete the project as soon as possible as a technology implementation. The organization did not want to waste cycles defining a good plan.
He then said, “Our technology is tough to use. It will never be a good fit for our business. I am just waiting for Artificial Intelligence to become mainstream and fix it.” I laughed, thinking silently, “Even AI cannot fix stupid.” The discussion got my juices flowing for a long day.
The Day
I was a featured speaker at a small event. The group’s goal was to drive process innovation through collaborative networking. The primary dialogue was on improving conventional models with AI engines. The group wanted to focus on models and engines, so the conversation was void of a discussion of outcomes.
I followed a professor from MIT. In his speech, he shared a case study on improving logistics costs in the face of variability through advancements in AI.
Introduced as a contrarian, I planted my feet and faced the audience. My presentation focused on the journey from cost mitigation to value creation. My message was AI cannot help if we are unclear on the desired outcome. Most supply chain leaders focus on cost reduction and are unclear on value-based outcomes.
I am no spring chicken. In my forty years in the profession, supply chain leaders have waxed eloquently about improving models and engines to reduce functional costs. I want to broaden the discussions to focus on policy, strategy, and rules automation, but most groups cannot help themselves. They want to keep discussing models and engines to reduce costs. (Hence the Facebook analogy.)
Companies speak about moving from a functional metric focus to managing corporate metrics, but this does not happen. Traditional processes are tethered to functional cost reductions. It reminds me of this picture from Facebook.
Let me give you some context. The man in the picture posed for yearbook pictures in the same clothes for forty years. The man aged, but the look did not. I feel that supply chain processes are analogous.
Figure 1. Facebook Analogy
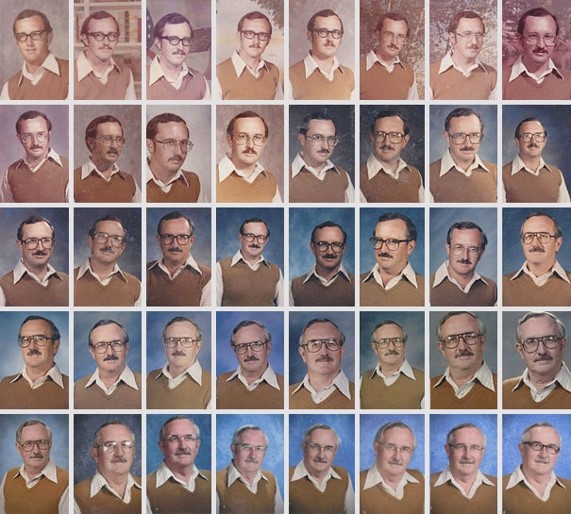
My research shows that functional cost improvements do not translate to operating margin improvements. Improving operating margin is important. Of the 114 balance sheet metrics tested in work with the statisticians at Georgia Tech, operating margin improvement has the highest correlation to market capitalization. (Higher than EBITDA, EBIT, Cost of Goods Sold, and Gross Margin.)
Mistakes
In my presentation, I highlighted mistakes.
- Supply Chain Performance Does Not Have Unlimited Potential. Companies implement technology projects, assuming that the supply chain has unlimited potential to improve. However, there are trade-offs, and an effective frontier bounds the outcomes. To advance, the company needs to focus on a step change to improve the outcome.
- The Efficient Supply Chain Is The Most Effective. This myopic approach assumes that the most efficient supply chain (lowest cost) is the most effective. Companies manage a singular supply chain even though there are five to seven distinct flows. Only 20-25% of supply chain flows can be managed efficiently. The larger and more complex the company, the smaller the impact. As a result, large companies underperform smaller regional players.
A Case Study
In my presentation, I treaded lightly; the Pfizer team sat in the audience. Pfizer ranks number 21 on the coveted Gartner Top 25 analysis, but the company underperforms the pharmaceutical industry in terms of operating margin, inventory turns, and Return on Invested Capital (ROIC).
I got my Pfizer booster that morning and I wanted to praise the team for rallying to the market crisis for COVID vaccinations, but I needed to make a point.
The Pfizer orbit chart, shown in Figure 2, tells the story. When we push the patterns of this performance profile into the regression model for market capitalization by employee, we find the team underperforms on value when compared to their peer group by 32%. Capturing this value will never happen by focusing on cost reduction based on functional metrics no matter how advanced the engines.
Figure 2. Orbit Chart Comparison of Pfizer to the Pharmaceutical Industry Performance
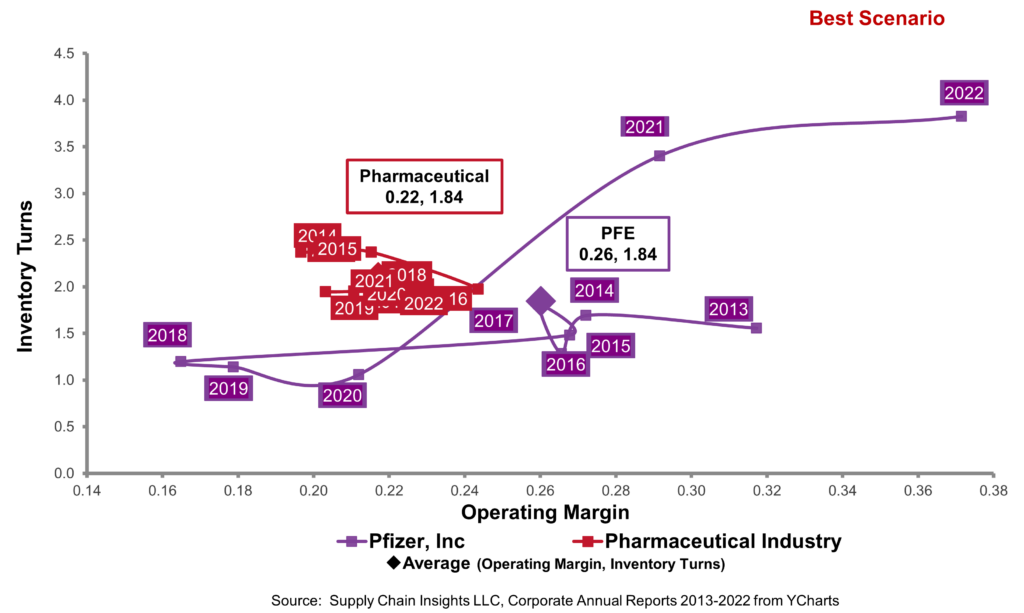
Summary
The supply chain has aged, but our discussions remain the same. The focus is on cost reduction, and we are unclear how to drive value. The discussions are the same even though new techniques offer promise. Let’s change the discussion to focus first on outcomes and then use new forms of analytics to improve value.
My fear? I believe that we will try to AI stupid.
My goal is to build models that enable the supply chain leader to engage the leadership team in a discussion of value. However, many teams need to reskill to talk the language of value-based metrics to meet this goal. The first step is to use network models based on our regression work with Georgia Tech. (We will have completed this work for all industries by November.)