Cloud. Descriptive analytics. Prescriptive analytics. Machine learning. Collaborative workflow. What-if analysis. I find, as I attend conferences, that the word ‘planning’ is bandied about, but few technologists dive into the details. We have lost the ability to have a discussion on the fundamentals. It drives a supply chain planning gal like me crazy. Here I share some insights on planning. For everyone trying to navigate new technology options, this is an important question.
A Look Back
The traditional world of supply chain planning managed inputs into a data model to drive outputs. While we can argue about which technologies have the best role-based usability and what-if analysis, the key to the selection of supply chain planning is the fit of the data model and the ability to drive an output that drives business value. A mistake that many companies make is a focus on implementation. Instead, it was about fine-tuning and model configuration. The goal should not be about speed to implementation. Instead, it should be about the “success of the model output” to drive value.
With the evolution of Enterprise Resource Planning (ERP) platforms and the zealous implementations of many business consultants, the basics of planning were lost. As companies focused on the hype cycle of the “integrated supply chain model,” the projects were large and complex. The focus by IT on implementation often left a business leadership team with a tool that they could not use.
Evolution Issues
Today, the client-server technology vendors are moving to cloud-based deployments. However, companies like JDA, Logility, Oracle, and SAP are hamstrung by software maintenance upgrades, promises to customers, and evolution. One of the largest blights in the market is the SAP evolution of APO and the lack of clarity of the roadmap. APO became the market leader despite the lack of functionality, and the IBP roadmap makes this worse.
In parallel, companies seeking a path forward are being deluged by “schema on read” technologies, cognitive computing, prescriptive analytics and machine learning which is largely making the traditional planning world obsolete. The traditional engines were based on linear optimization. As supply chains grow more complex (with an increase in items, and lumpy demand due to globalization), they are increasingly becoming more nonlinear. This requires deeper modeling and the use of channel data. Linear optimization assumes a normal distribution of data. For the distributions that are not normal, machine learning and cognitive computing are gaining traction. (Credit for this image goes to ToolsGroup. I liked their image.) The point is that the area in blue represents high-volume items that typically represent statistics based on a normal distribution. Traditional supply chain engines assumed a normal distribution which is increasingly becoming a smaller data set.
Figure 1. The Long Tail of the Supply Chain Requires Deeper Engines Using Nonlinear Optimization
In addition, as business becomes more complex, the assets are more utilized and the business has more manufacturing constraints. Constraint-based supply requires deeper modeling of manufacturing and network modeling. The business teams struggle to keep skilled planners. There is a need for a connection from the core planning world of supply planning to business leadership capabilities with mature collaborative workflows and “what-if” capabilities. In a global organization, there are typically different needs for three core roles as shown in Figure 2. Core planners need deep models while business users need “what-if” capabilities connected to the models along with a “collaborative workflow.” The core planners need deep modeling and access to clean master data. This is an issue for most companies. Traditional Advanced Planning focused on core planners but not usability by business leaders and senior management. Technologies like Anaplan, Halo and Kinaxis improve visibility for these key roles.
Figure 2. Planning Requirements Vary by Role
Technology Acceleration
As we move forward, there is a lot of discussion on descriptive analytics and the ability to get data. There is also a lot of hype on “connected planning.” However, I scratch my head. How can we have connected planning if the organizations are not aligned, and do not have a balanced scorecard that crosses across the company to drive functional alignment?
Figure 3. Organizational Alignment
Collaborative sales planning introduced in the last decade increased error and bias. Asking sales for a forecast is vastly different than sensing the market based on channel data. To win, companies must get aligned cross-functionally on the role of the budget, and drive alignment on the strategic plan.
The technology market is moving in two directions (deeper modeling through cognitive computing and greater visibility through descriptive analytics). In this evolution, confusion reigns. This includes hands-free planning with “schema on read” machine learning and easy-to-use descriptive analytics with easy “what-if analysis” that is not anchored with deeper modeling. These are polar opposite pulls that actually need to be brought together. With the confusion on the SAP migration plan for 2025, more and more companies are in the market looking for solutions. The frenzy is high and the increased confusion on the technology options increase the number of questions. As you try to navigate the market don’t forget the basics.
Planning Basics
Whenever someone uses the term “real-time planning” raise a red flag. Planning is not, and should not be, real-time. Attempting to do planning in real-time will add nervousness and error into the planning processes. Don’t confuse sensing and translation with planning. Avoid any process that is described as real-time planning.
Planning time horizons exist for a reason. They are the natural boundary for global versus local planning governance. The best teams define governance and are clear on time horizons. There are natural processes which require a tactical horizon for procurement buying transportation sourcing, and Sales and Operations Planning (S&OP). In the tactical time horizon, shifting and aligning manufacturing loads across factories is critical. If you are a regional or a small company, tactical planning is not as critical. The best companies are great at strategic network design and translate this to a global planning team that sets the network for a longer period of 18-24 months. Being good at tactical planning is essential for managing costs. The shorter-term horizon of 0-12 weeks benefits by faster planning planning cycles but short-term operational planning is not sufficient to manage the supply chain. It is best when complemented by strategic and tactical planning. In addition, cognitive computing and the redefinition of workflows for “multiple ifs” to “multiple thens” is transformational for the redefinition of Available-to-Promise (ATP), allocation, and inventory management workflows. Don’t make the mistake of eliminating time horizons. Use new technology options to improve hand-offs and visibility and reduce planning batch cycles.
Test but verify. The use of deeper engines and workflows can improve outcomes, but only if we test and learn. Never treat a planning project as an implementation. Test. Learn. Evolve. So often, companies forget to configure the system and test the output of the planning engines.
Sales-driven processes are not market driven. Marketing-driven processes are not market driven. Market-driven processes are outside in starting with the customer and translating data back into the organization as a usable demand signal. The best results happen when the focus is on the market, using channel data, not on functional planning. Aggressively build outside-in processes.
As you consider the options, it is important that you ask the question “What is planning?” Don’t accept historic views of planning limitations, but don’t forget the basics. And, if you have questions, let us know.
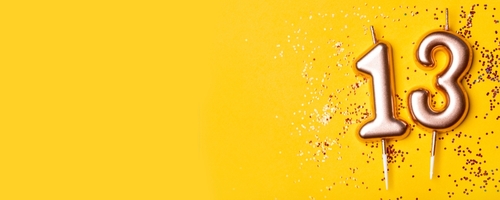
Thirteen Years a Blogger
Thirteen years. 600 posts. Where does time go? For over a decade, since founding Supply Chain Insights in 2012, I have pounded the keyboard, asking